RecommendMail Facebook LinkedIn
- Digitization
- Smart Manufacturing
- Mobility
- Health & Science
Photonics meets artificial intelligence
Three examples of how photonic technologies can be combined with artificial intelligence to enable users to reach their goals faster and more reliably.
, Katrin LauterbachSee, recognize, assign – and do so as error-free as possible and in real time. The goal of many processes based on visual image recognition may sound as simple as this – whether for quality checks, evaluation of samples or the allocation or assignment of permits and rights, for example for access checks.
If artificial intelligence is added, this usually means that the speed increases or – in the best case – also the detection rate. It is up to you what exactly is recognized and evaluated as this will be based on your business requirements, because there are (almost) no limits to the image processing systems.
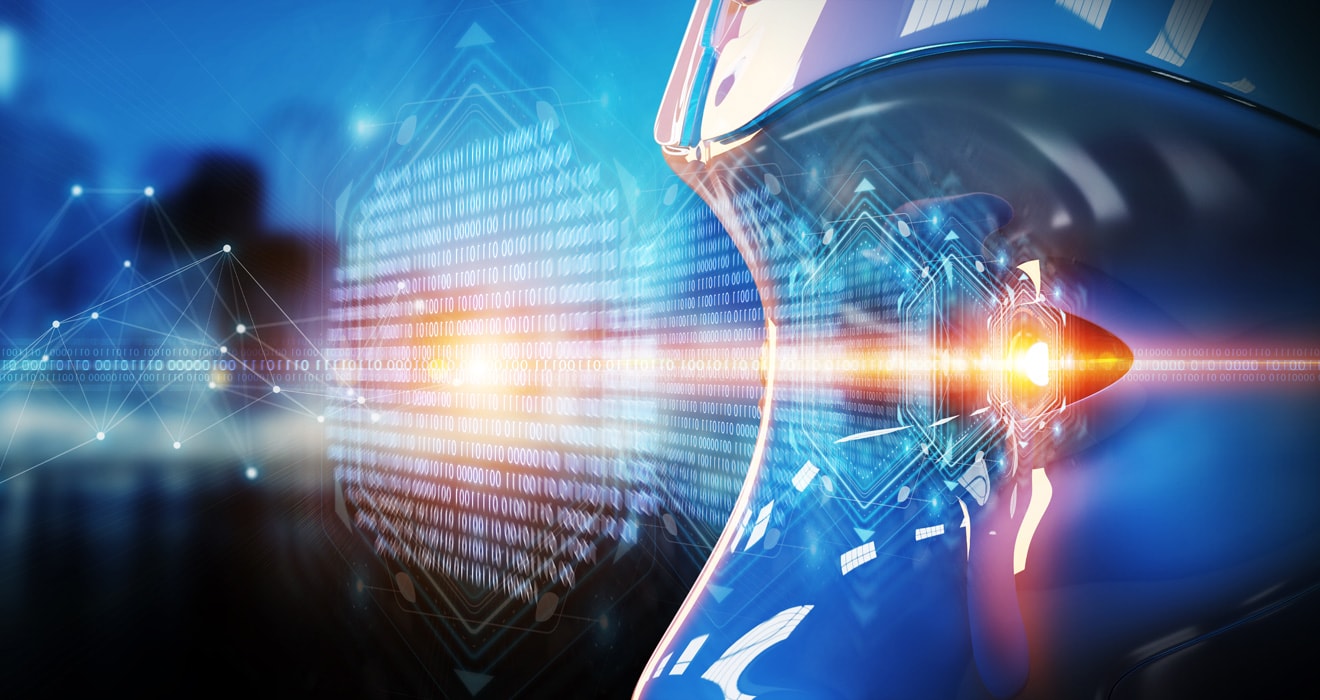
Why do photonics affect many applications of artificial intelligence?
They enable AI to "see" in the first place and thus the analysis. The initially invisible is made visible in complex test sequences with the help of highly sensitive sensors and camera systems. Imaging techniques provide high-resolution and high-contrast images. In combination with deep learning algorithms, even more information can then be extracted from images. This leads to improved results in a wide range of applications in research and science, industrial manufacturing, in healthcare and the public sector.Example 1 – More reliable analysis of samples in medicine by using artificial intelligence
In recent years, the use of artificial intelligence in the medical sector has become increasingly promising. Compared to other industries, however, some restrictions apply that are intended to protect patients in the best possible way It was therefore an important step for the medical industry when the US Food and Drug Administration (FDA) founded a specialist team in 2018 to review and approve the use of deep learning algorithms for medical technology. But what are the benefits of AI for healthcare?
Imagine you have a microscope with a medical sample on the slide in front of you. Transferred to the doctor's screen, the image of the sample has an incredible 15 gigapixels – an enormous number of pixels ensure high image quality. And it is precisely in this large number of pixels that you are looking for, for example, a conspicuous but tiny deviation, a so-called micro-malignancy, which can, for example, be an indication of a tumor disease. This is usually only 300 x 80 micrometers, i.e. only 0.3 x 0.08 millimeters. For comparison, normal human hair is less than 100 micrometers thick.
What sounds like a challenging diagnostic task is an important core skill in a pathologist's day-to-day work. On average, pathologists take about two minutes to analyze a sample under the traditional professional microscope. And this is precisely where AI can help and speed up this work process.
Together with a search engine company, Jenoptik developed a microscope camera that allows clinicians to work more precisely and efficiently. Markings show pathologists which areas they should examine more closely – that is, areas of the samples they should focus on. Jenoptik's microscope camera, augmented with AI software, can highlight specific areas on the samples that are of high interest for disease detection. This is because the system has learned from the determination of previous samples which patterns each micro-malignancy has and displays these in real time.
Example 2 – Artificial intelligence improves quality assurance in series production
In production processes, a decisive factor for the efficiency and quality of the added value is keeping the number of rejects as low as possible in order to bring the proportion of error-free production parts, the yield, close to 100 percent. For example, high-speed and inline inspection cameras are used in many production areas to detect defects in surfaces or shapes during production. High-resolution camera systems check the production units for errors and deliver precise and high-contrast images. Here, too, the combination of visual image processing with artificial intelligence increases the quality of error detection. In addition, errors can not only be detected more reliably, but can also be classified more precisely – which in turn simplifies the evaluation of suitable adjustments or countermeasures.
For example – welds and screw heads:
Quality control of welds can be very complex. This is because even welding points that look good at first glance show differences, which is why product defects are often difficult to identify. It is similar, for example, in the quality of screw heads. Also, only a very small quantity of thousands of parts produced are defective. Distortions, reflections or dirt during production also pose an additional challenge for the imaging inspection procedures.
Thanks to neural networks and a deep learning algorithm, cameras can quickly and unambiguously identify whether production parts meet the defined quality requirements. Such analytical systems are trained with large datasets of error-free parts. In this way, the system learns to detect even the smallest deviations and to separate the "good from the bad parts" within the production process. Thanks to photonic solutions, the algorithm is based on high-resolution images. Combined with deep learning algorithms, these provide more accurate results in the analysis of images. If new parts are produced that are also inspected, the algorithm autonomously recognizes whether there are anomalies or not – and continues the learning process. Immediate decisions on corrections in the production process can be made by identifying errors more quickly and systematizing them.
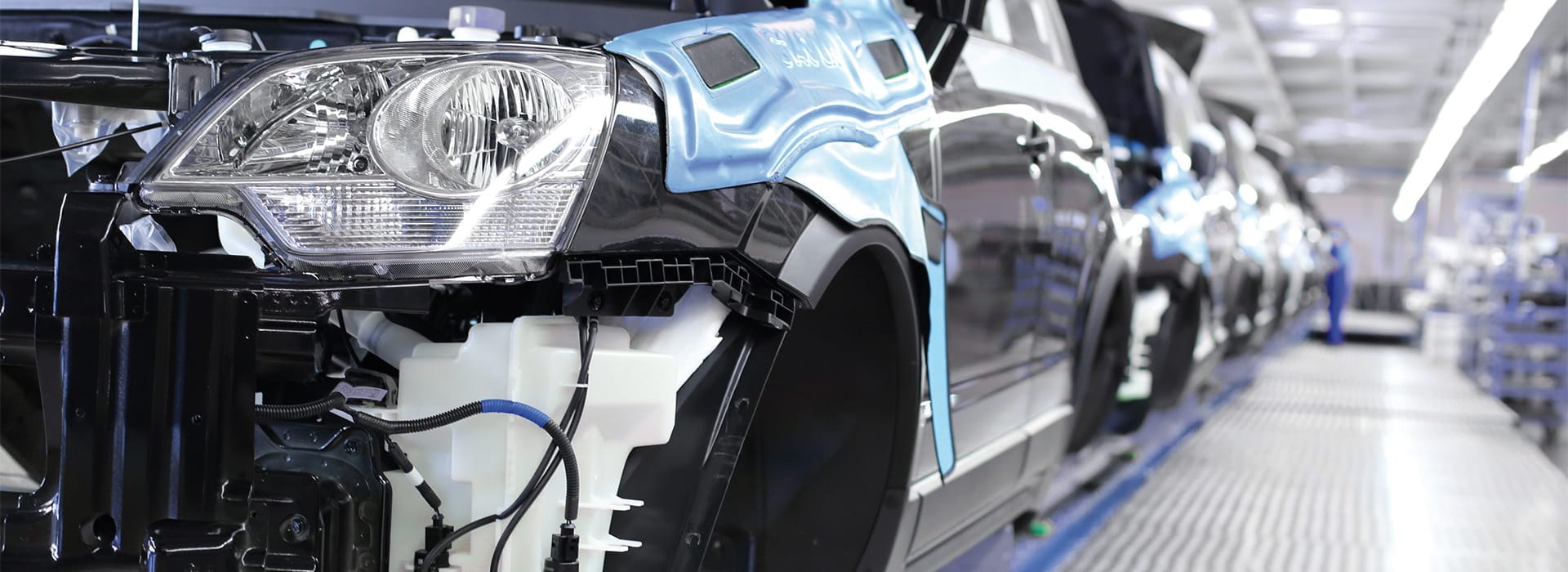
Example 3 – Number or license plates can be read correctly even under the most difficult conditions thanks to artificial intelligence
The use of artificial intelligence also offers significant advantages in the area of road safety, for example in automatic number plate recognition systems (ANPR; aka automatic license plate recognition, ALPR), which are used for access rights as well as speed controls.
The challenge is to correctly identify the number plates even in difficult conditions, such as bad weather conditions, darkness or weak lighting. The AI system recognizes patterns and recurring elements, i.e. it "understands" what the number plates convey about the country of origin of the vehicle, , thus increasing the read rate accuracy. In principle, the software can also classify vehicles into car, truck, bus or motorcycle
The AI software is integrated directly into the traffic cameras, so no additional equipment or installation is required. And the integration of ANPR into police information and search systems, for example in the United Kingdom, ultimately simplifies police work and contributes to making roads and communities safer.
Hundreds of AI based ANPR cameras are currently operating with Police forces across the UK. With their superior read rate accuracy, they help to keep communities and roads safer because they reliably and rapidly identify vehicles of interest, allowing the police to carry out appropriate interventions.
The examples show that self-learning systems are systematically finding their way into everyday life and are making processes faster and more reliable. Nevertheless, the machine will not replace people – at least not in the foreseeable future. Artificial intelligence is still created by humans and it depends on the quality of the sample data with which it has been trained.
Do you need camera systems combined with learning algorithms to improve your processes, quality assurance and analyses in medicine, production or mobility?
We look forward to your message!
Artificial intelligence, machine learning, deep learning – what is actually what?
The more abstract term artificial intelligence (AI) covers a large number of technologies and measures that are used in a wide range of areas. Overall, it is about transferring human decision-making structures to technical systems.
AI includes machine learning processes, among other things. These are based on predefined algorithms that analyze data, learn from it and then make decisions. In short, they learn from experience. For this purpose, artificial neural networks can be used, which was the starting point for modern deep learning.
Deep learning is a subfield of machine learning concerned with algorithms inspired by the structure and function of the brain called artificial neural networks. The term “deep” comes from the fact that the learning process involves many layers of networks (three or more) which otherwise is also known as deep layered networks or deep neural networks. Hence, due to usage of deep layered neural network for machine learning approach this is termed as deep learning.
Deep learning algorithms are similar to human neural networks and in the last few years it has achieved exponential success especially in the field of Computer Vision and NLP (Natural language processing). In fact deep learning algorithms already outperform humans in certain tasks (e.g. mitosis detection).
With appropriate data, deep learning algorithms and sufficient training, machines can understand patterns in data and generalize the knowledge learnt and apply it to new data with very high accuracy and reliability. Deep learning algorithms are also capable of transferring knowledge from one task to another. Deep learning at Jenoptik ranges across applications starting from manufacturing with sorting defective parts out in production lines to healthcare sector to evaluate for anomalies in medical specimens. And it is just the beginning of new era of AI.
About Katrin Lauterbach
Katrin Lauterbach is part of Jenoptik’s Corporate Communications and Marketing department. As a qualified journalist, she has always had an aptitude for writing, among other skills. She is passionate about translating technology and business into understandable texts. After her internship and business studies, she started at Jenoptik in 2000, then worked for Bosch and Sparkasse, a German bank group, and ultimately returned to Jenoptik in 2016. The focus of her work is currently the expansion of digital media.